Exploring How Artificial Intelligence Can Potentially Foster Environmental Conservation
AI, the silent force of sustainability, unlocks unprecedented potential for environmental conservation—a powerful ally in safeguarding our planet for future generations.
Embark on a profound journey into the realm where cutting-edge artificial intelligence (AI) collaborates seamlessly with the ongoing efforts in ecological conservation. This exploration delves into the transformative synergy between AI technologies and environmental preservation, shedding light on the remarkable ways in which these advanced tools are actively utilized. Discover the previously unimaginable applications of AI, from revolutionizing wildlife monitoring to optimizing afforestation initiatives and leveraging predictive analytics for climate change assessment. Witness the unfolding narrative of how AI, as a dynamic ally, is reshaping the landscape of ecological conservation, introducing unprecedented possibilities for safeguarding the environment and fostering a sustainable coexistence between technology and nature.
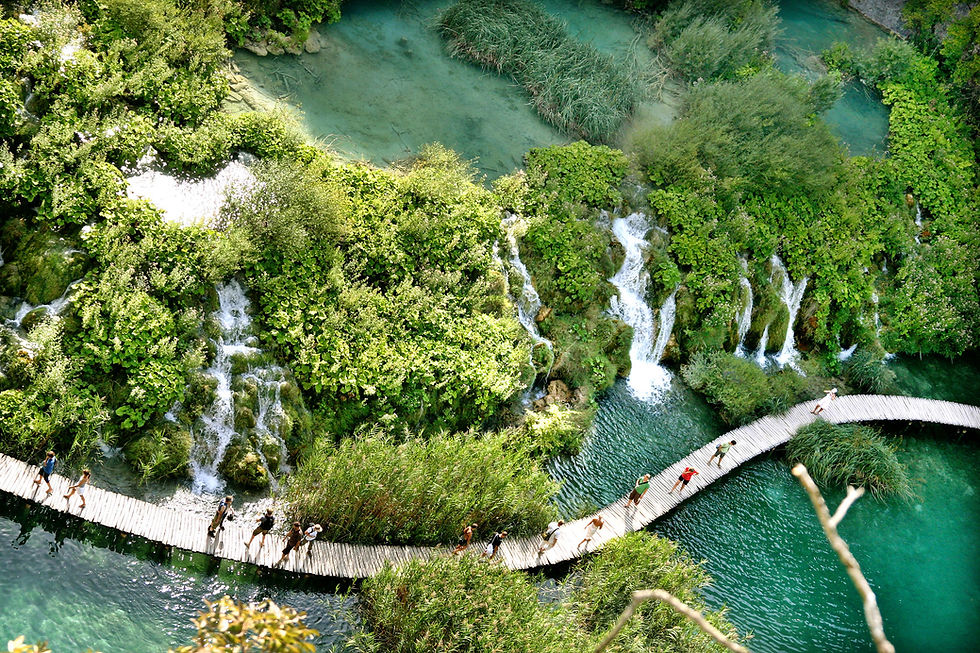
This blog post illuminates the transformative impact of artificial intelligence on ecological conservation, showcasing how innovative technologies are becoming essential allies in our collective efforts to protect and preserve the environment.
At the crossroads of technological advancement and environmental stewardship lies a profound convergence – the fusion of artificial intelligence (AI) with the multifaceted endeavors of ecological conservation. This dynamic synergy is revolutionizing the way we approach the preservation of our planet's intricate ecosystems, unveiling a realm of transformative applications that were once confined to the realm of imagination.
AI's prowess in data analysis, machine learning, and pattern recognition is proving invaluable in the monitoring of wildlife populations. From analyzing satellite imagery to deciphering acoustic recordings, AI algorithms are enabling conservationists to track species movements, identify habitat degradation, and detect illegal activities with unprecedented precision.
In the domain of afforestation, AI is optimizing strategies for replanting and restoring degraded landscapes. By analyzing soil conditions, climate patterns, and land-use data, AI algorithms can pinpoint optimal planting locations, species selection, and resource allocation, maximizing the success of afforestation initiatives.
As climate change looms, AI's predictive capabilities are proving indispensable in assessing the potential impacts on ecosystems and developing proactive adaptation strategies. By analyzing vast datasets of climate models, historical observations, and ecological parameters, AI algorithms can predict shifts in species distribution, changes in precipitation patterns, and the likelihood of extreme weather events.
The integration of AI into ecological conservation is not without its challenges, requiring careful consideration of ethical implications, data privacy concerns, and the potential for bias in AI algorithms. However, the potential benefits of AI in safeguarding our planet's biodiversity and fostering a sustainable future far outweigh these concerns, making it an indispensable tool in the arsenal of conservation efforts.
As AI continues to evolve, its impact on ecological conservation will undoubtedly deepen, shaping the way we monitor, manage, and protect the natural world. This symbiotic relationship between cutting-edge technology and environmental stewardship holds the promise of a future where humanity and nature coexist in harmony.
Harnessing the prowess of artificial intelligence (AI), innovative strides are being made in ecological conservation. AI is dynamically employed in wildlife monitoring, where advanced algorithms and machine learning models revolutionize tracking and protection efforts. Autonomous eco-drones equipped with AI soar through the skies, surveying ecosystems and detecting environmental changes. Smart afforestation initiatives benefit from AI's capability to analyze soil conditions, climate data, and biodiversity requirements, optimizing tree planting for more sustainable ecosystems. Predictive analytics powered by AI contribute to a deeper understanding of climate change trends and potential impacts. In precision agriculture, AI fosters sustainable land use by optimizing resource utilization and reducing environmental impact. AI extends its reach to become the digital guardians of oceans, deploying underwater drones for marine life monitoring and protection. Lastly, crowdsourced environmental data analysis is enhanced by AI algorithms, offering valuable insights into ecological trends through collective contributions.
These applications collectively represent the transformative influence of AI in shaping a harmonious future for ecological preservation and sustainability.
AI-Powered Wildlife Monitoring
Autonomous Eco-Drones
Smart Afforestation
Predictive Analytics for Climate Change
Precision Agriculture for Environmental Sustainability
Digital Guardians of Oceans
Crowdsourced Environmental Data Analysis